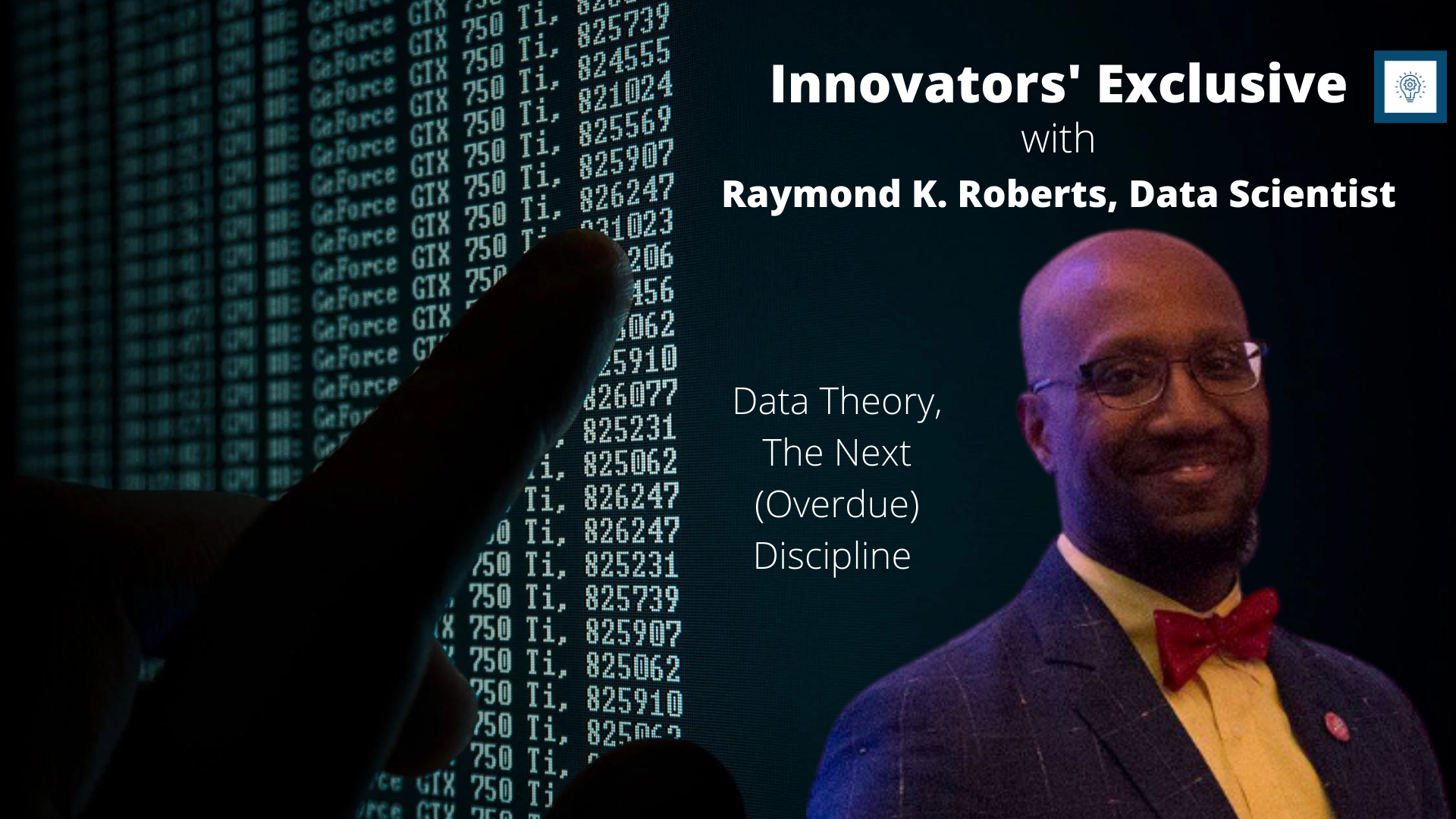
Data Theory, The Next (Overdue) Discipline
By Raymond K. Roberts
Digital Transformation. You have heard the term and sales pitches associated with it, so we can skip that. Let’s dive directly into what Author David Tenner may hopefully agree with me in identifying an “efficiency paradox”. Remember learning about how every decision has a cost in Econ 101? Well an efficiency paradox is the cost we pay for when leveraging the latest and greatest technology, vendor product, tool, or service. There are familiar conversations surrounding Digital Transformations and data initiatives in general. Those conversations include, but are not limited to:
- Conversations about how over 80% of human resourcing time on a data & analytics project is spent collecting & standardizing master data, connecting it to reference data, and aggregating/enriching it. Is this a benchmark that we can’t change? For firms generating most of the data of interest, can something be done proactively through business leadership guidance (governance) and data engineering and design efforts (provenance) to increase the amount of time spent doing analysis in support of applying said analysis?
- Conversations about how data initiatives do not respect the traditional business unit silo rules (cross functional efforts). But what rules or domains (if any) does data respect?
- Conversations about the definition of data quality after a reactive application attempt, rarely proactively before (past missingness, granularity, relevance & correctness). Are there universal or “business case agnostic” metrics to measure data by?
- Conversations about collecting current business cases, thus taking a static snapshot of the situation. Are there ways to engage the dynamism of the situation over time? Is there frameworks and methodologies to engage the “certainty of uncertainty”? Instead of building robust solutions, can we build Author Nassim Taleb’s antifragile processes that improve the overall position when engaging something never seen before? Can “built to spec” be the process of engaging problems rather than simply the current solution to the current problem?
The more deliberate journeys and more desirable destination for such conversation I would like to term as Data Theory, and the application of Data Theory, Data Strategy. While Data theory is not a completely new term, a quick online search will find most of the focus around exploratory data analysis (EDA) and confirmatory data analysis (CDA). EDA includes but is not limited to univariate, bivariate, relationship analysis of a dataset. CDA is the investigation of whether what we say is happening is supported by the records persisted in the data regarding what happened. The problem however is that the processes areas of “doing” data has a much wider scope. The image below shows us six process areas for data.
The 6 Data Process Areas |
|||||
|
2.) Generation |
3.) Collection & Standardization |
4.) Aggregation (Enrichment) |
5.) Analysis |
6.) Application |
EDA and CDA only cover at best processes 4.Aggregation and 5.Analysis. There is much more support for process 5 through the statistics and data science fields, and also in the application process through the visual communication field. But where is the guidance for generating data in a way that aligns with business needs both known today and unknown tomorrow? Where is our guidance for knowing what rules to make for data, making them, and ensuring those rules are being adhered to? When you are weighing your options between building solutions or buying from vendors, what is the guidance for ensuring your overall strategy and metrics that you are using to make the decision are valid? I mean…..other than popular support or sponsorship by a power-broker or business leader? How is merit evaluated across the six data processes?
Data Theory is the foundational education, philosophies & methodologies that endeavor to remove as many of the proxies between the data and humans as possible. Removing the business units, the custom metrics, current problems at hand. Applied Data Theory produces merit based, process-centric deliverables that align with Data Theory concepts. Data Strategy is the design, build and deployment of the work instructions, tools and solutions. Items that not only weather the current times but thrive in them. Data Theory is the “what”. Applied Data Theory or Data Strategy is the “how”.
Larger organizations have been fighting with the Army that they’ve got, that is, the existing implicit data stewards, engineers, and architects to fight this war. I can tell you that across many organizations these have been some of the most amazing and intelligent individuals I have ever had the pleasure of working valiantly leading the charge on implementing and actioning the “how”.
Early 19th century French economist Frederic Bastiat wrote on the fallacy that because someone has spent their life working for, earning, and handling money, this should translate to their ability to “know” money. Think about it. Someone has been working since they were 10 years old and thus have over 50 years of employment history. Is this enough information to hire them as your money manager? Of course not. So why do we assume a long career in using data translates to an individual being the right person to be your “data manager”?
William Gibson is attributed with the quote “The future is already here. It is just not evenly distributed yet.” I have seen the future. The future is in the form of explicit roles existing under Chief Data Officers with titles such as “Data Strategist” with prefixes such as “Chief”, “Principle” or “Staff”. Individuals tasked with both the strategic concern of managing data as an asset through its entire lifecycle, but within this corpus of individuals, tasking with the operational tactics of designing processes and work instructions on HOW to be strategic about data in an exponential-rate-of-progress world.
I argue that Digital Transformations & Progressions cannot proceed faster than the rate at which:
- people can be educated to design, construct, and build platforms and processes
- people can be educated to operate and maintain platforms and processes
Furthermore, while I see this as a new explicitly named discipline, I see it pulling from the existing interdisciplinary rainbow no different than how Data Science is doing. Philosophy, biology, behavioral economics, and physics for a short list will all be required to bring this field to full yield.
The future begins with the data but will always be about the people.
Data First, People Always.
Interested in learning more about Data Theory and its applications? Contact the University of Wisconsin Parkside, Kenosha at their Smart Cities Innovation Center for more information.